Service Optimization of Novel Distributed Energy Regions (SONDER)
Project duration
Start: 01.01.2019
End: 31.03.2023
Demo sites
Vienna (Austria)
Lulea (Sweden)
Arbon (Swiss)
Project costs
2.273.424,75€
Call
ERA-Net SES Joint Call 2018 RegSys
Project Overview
Local and regional energy systems are expected to become essential partners for distribution system operators and significant contributors to energy system stability. Typically, power peaks can be buffered by the grid, but the unpredictable nature of renewable energy sources, the unexploited efficiency of gas, electricity, and biofuels in operating buildings, and electric vehicles concurrencies lead to unknown correlations. There are no strategies available for communities to manage distributed energy resources, help optimizing local efficiency, pool, orchestrate, and command flexibilities in an increasingly digital world, and offer that flexibility on the markets. Building on existing knowledge of national and regional projects, together with stakeholders in NGOs, industry, and ESCOs, we investigate novel services for communities to generate, consume, provide demand response, and storage in a pan-European setting, including smart cities, residential, industrial, and data center users.
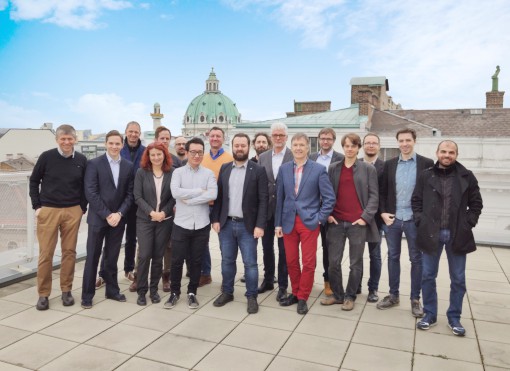
Main Objectives
- Identification and definition of specific need owners and their needs with respect to local/pilot situations. These can serve as point of departure for future work.
- Development of a microgrid model which includes renewables resources (Solar, Wind and hydro), centralized power generation and flexible services including centralized storages and data center, with the aim to run case study scenarios to validate the research results from LTU and other academic partners from the other regions in the project.
- Integrate the flexible services model based on the configuration at SICS ICE into the microgrid model load model. Different configurations can be point of departure for further scientific investigation and research.
- Develop a VPP model of the microgrid (Including individual actors within the microgrid) using industrial standard based data models (I.e. IEC 61850) and the data infrastructure that is required for interactions between the various actors in the microgrid. This contributes towards addressing the horizontal communication interoperability between the aggregated actors within the VPP.
- Design and develop a general graph-based framework for sensor data streams able to model data at different levels (e.g. from a single household to an entire regions). Provide a methodology and tools to enable an online, lifelong learning for designed predictors so that they will be able to track time evolution in the grid.
- Develop a machine learning-based short- and mid-term predictors able to exploit the graph-based data representation of the input streams.
- Development of a cost matrix for, assessing the performances of benchmarks models, the state-of-the-art solutions in load forecasting and our novel framework.
- Develop a data translation tool that analyze market communication (Energy market signals with EDIEL or CIM as the possible data models) and translate these through abstract terms to various technical protocols (controllable signals for renewable resources and other actors). This contributes towards addressing the vertical communication interoperability which allows the market signals to be directly interpreted by the device level actors (I.e. the renewable resources).
- Demonstration in a co-simulation framework which interfaces the microgrid simulation model with the energy market. This is to firstly, validate the horizontal and vertical communication between the various actors. Secondly, a case study scenario will be tested to demonstrate how market incentives can be applied to incentivize data centers to take part in demand and response activities. This platform is also beneficial for the other academic partners in the project as a means to validate their research results.
- Provide a sensitivity analysis for future scenarios in order to assess the impact of new situations such as the adoption of new PVs, storages, EVs (electric vehicles), renewables or any other new load/production profiles in a particular location. Assessment of the grid flexibility to adapt to new situations avoiding expensive infrastructure upgrades.
- Increased grid flexibility potential enabled by advanced control of flexible services algorithms based on novel machine learning prediction engine and its assessment w.r.t standard control algorithms. Scientific exchange through workshops or conference discussions on the learned insights.